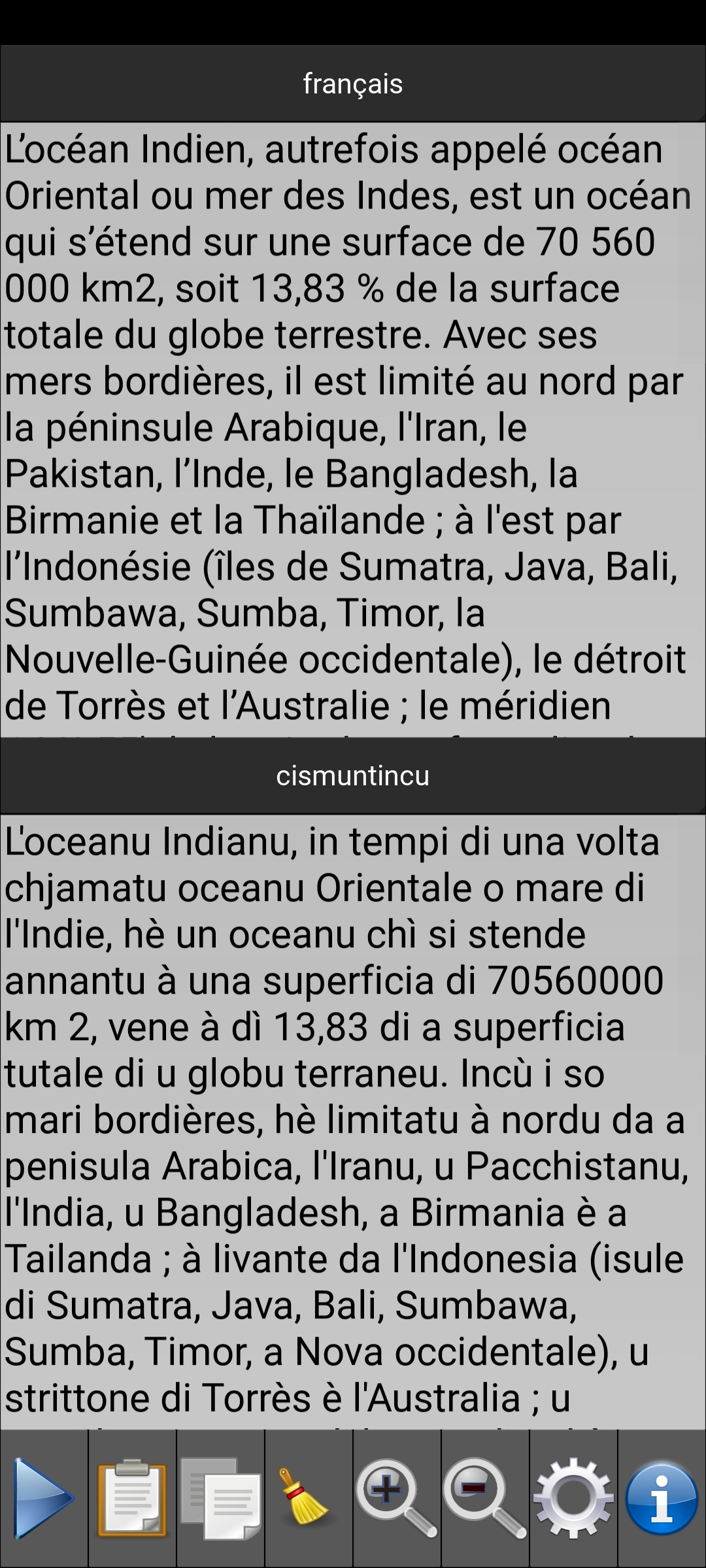
The Traduttore corsu application translates texts from French into Corsican. Respecting the polynomic nature of the Corsican language, the translation is carried out in one of the three main variants of the Corsican language: cismuntincu, sartinesu, taravesu.
The performance of the Traduttore corsu application is regularly assessed by means of an open test involving the translation of a pseudo-random text. The test involves translating the first 100 words of the “article of the day” from the Wikipedia encyclopedia into French. Currently, the software achieves an average score of 94% on this test.
Unlike machine translation software based on statistics or translation corpora, Traduttore corsu is based on the application of rules (grammar, disambiguation, elision, euphony, etc.). There are several reasons for this choice:
- No elaborate French-Corsican corpus currently exists
- Such a choice allows better control of the artificial intelligence used and traceability of the translation.
A number of options are available:
- Increase or decrease the size of characters displayed in the text to translate and translated text areas
- Paste text into the text-to-translate area
- Delete the text area to be translated
- Change the language of the application interface: Corsican (in one of the three variants cismuntincu, sartinesu or taravesu), English, French, Italian
- Choose between separate (e.g. “manghjà lu”) or grouped (e.g. “manghjallu”) writing of Corsican.
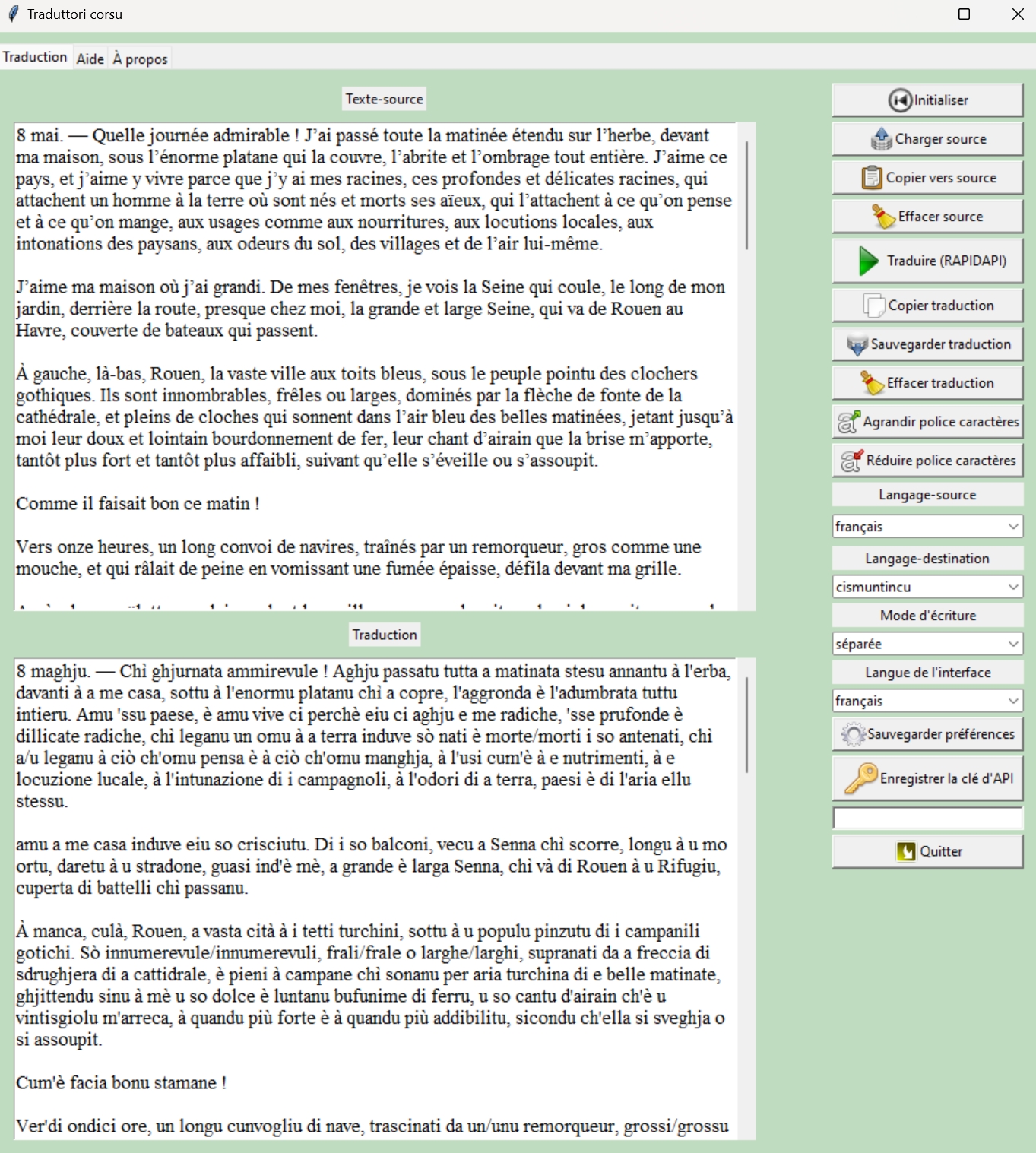
The free version lets you translate texts of limited length. The professional version can translate texts of any length.
Disclaimer: Translations resulting from the Traduttore corsu application are provided “as is”. No warranty of any kind, expressed or implied, but not limited to warranties of merchantability, is provided as to the reliability or accuracy of any translation made from the source language into the target language. In no event shall the author be liable to the end user for any claim, loss, damage or other liability, cost or expense (including litigation costs and attorney’s fees) of any nature, arising out of or in connection with the use of this translator.